Why Home Care Workers?
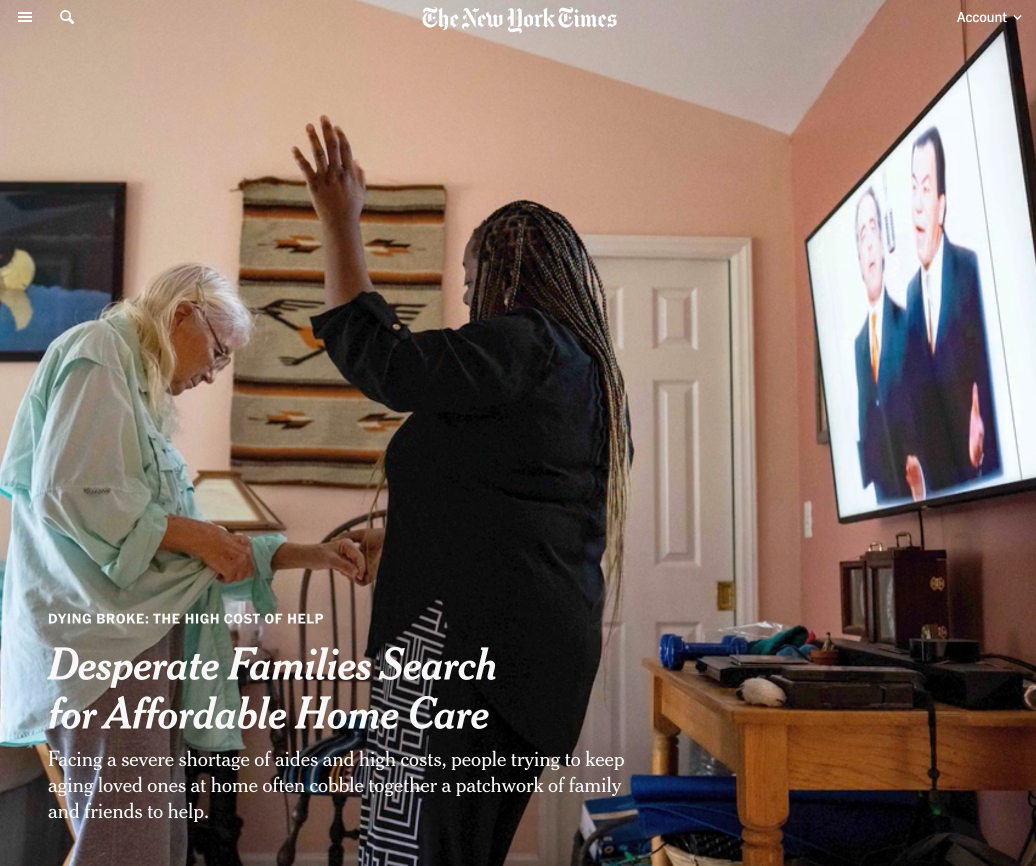
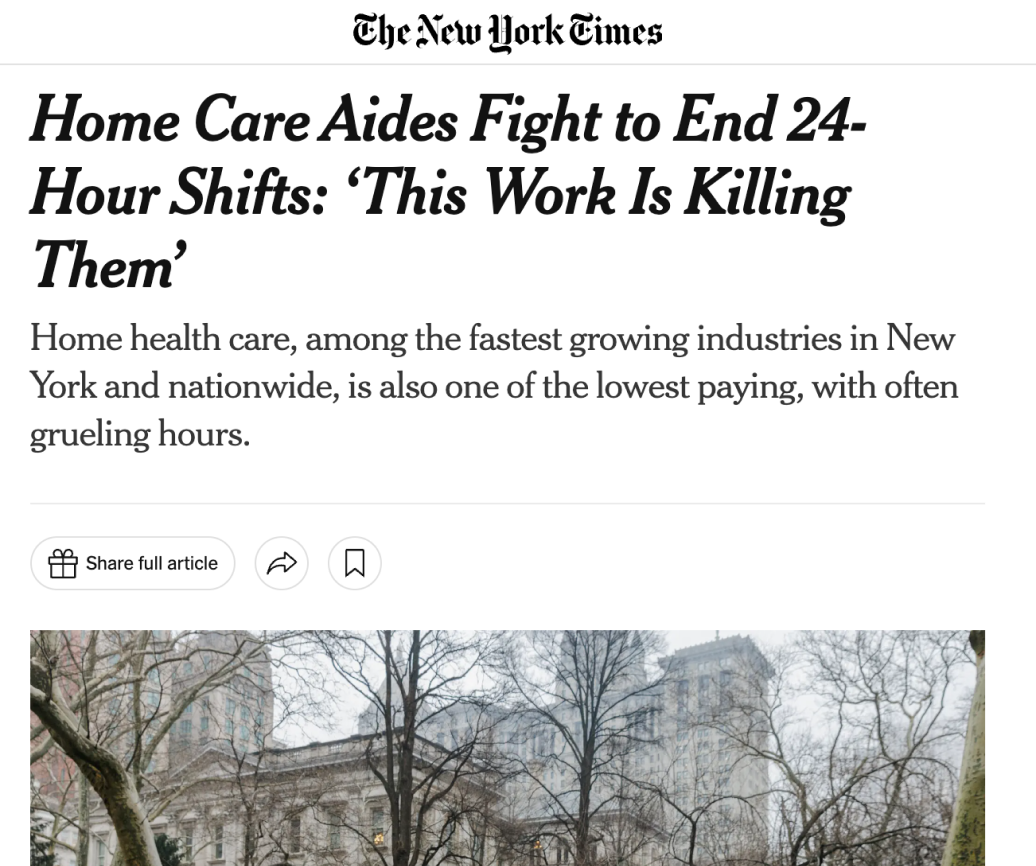
Home Care Workers (HCW) are an important group of frontline healthcare workers that deliver at-home care to older adults to enable them to age in place. Research has shown that HCWs, primarily consisting of Black and Latina women, are often overlooked and undervalued. They work in isolated conditions, are paid low wages and often experience stress and burnout.
With the aging population, the demand of home care workers is increasing. There is a pressing need to support these workers.
I'm advised by Dr. Nicola Dell and PhD Ian René Solano-Kamaiko, as part of the Initiative on Home Care Work at Cornell University.
With the aging population, the demand of home care workers is increasing. There is a pressing need to support these workers.
I'm advised by Dr. Nicola Dell and PhD Ian René Solano-Kamaiko, as part of the Initiative on Home Care Work at Cornell University.
Safe and Trustworthy AI

Second author for accepted paper at CHI 2025.
The ACM (Association of Computing Machinery) CHI conference on Human Factors in Computing Systems is the premier international conference of Human-Computer Interaction (HCI) and one of the top ranked conferences in computer science.
The ACM (Association of Computing Machinery) CHI conference on Human Factors in Computing Systems is the premier international conference of Human-Computer Interaction (HCI) and one of the top ranked conferences in computer science.
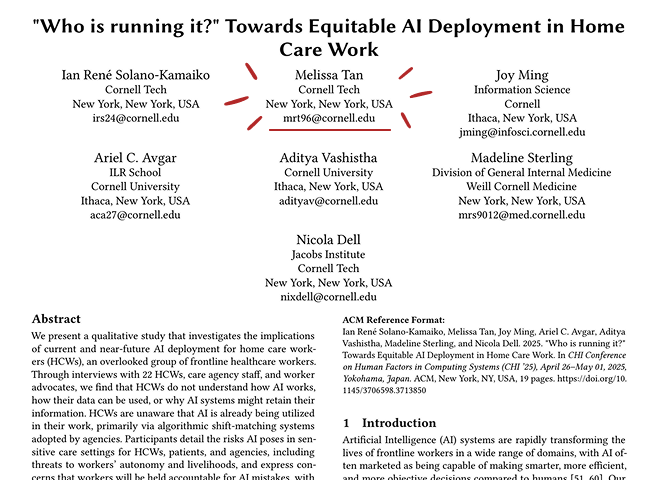
Qualitative Study on AI Deployment in Home Care Work (read here)
Summary
Expand for a pictorial overview of the AI study.
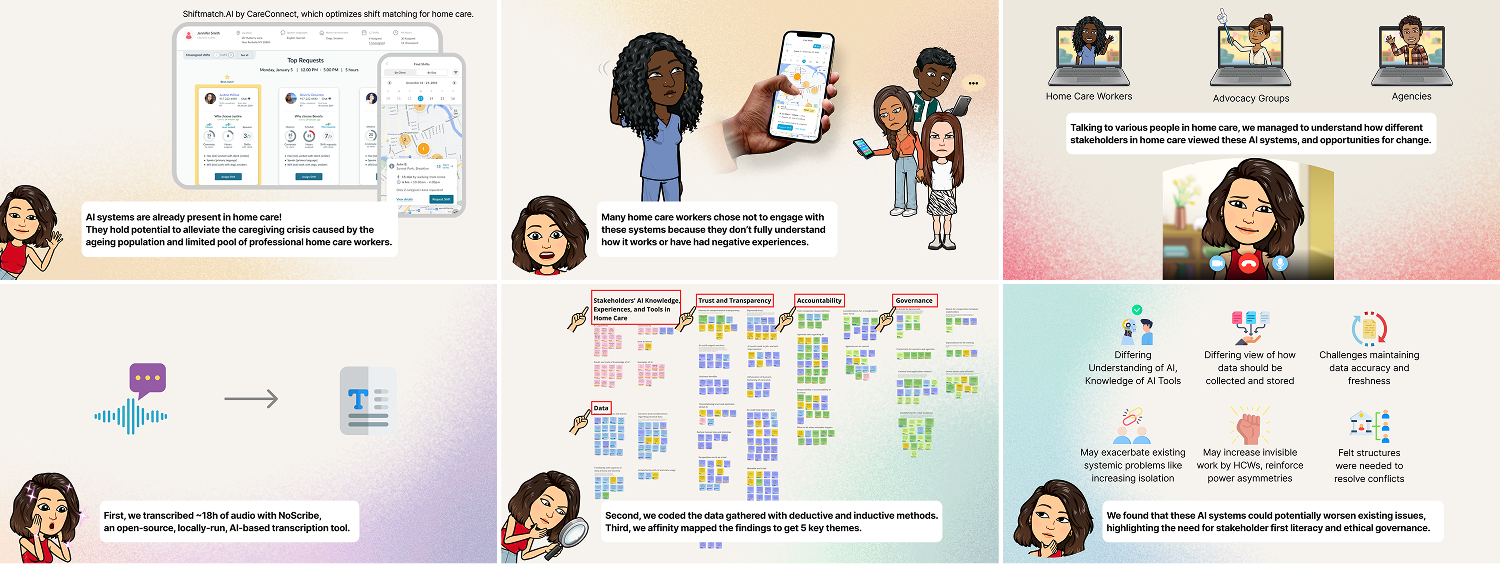
Story behind the AI work.
Problem
AI systems are rapidly transforming the lives of workers in a wide range of domains, healthcare included. With the rising demand for home care services following an ageing population, AI poses a potential to improve efficiency and reduce costs, helping to ameliorate the caregiving crisis.
Research Question:
What are home care workers, home care agencies, and unions' perceptions and understanding of AI use in the home-care industry?
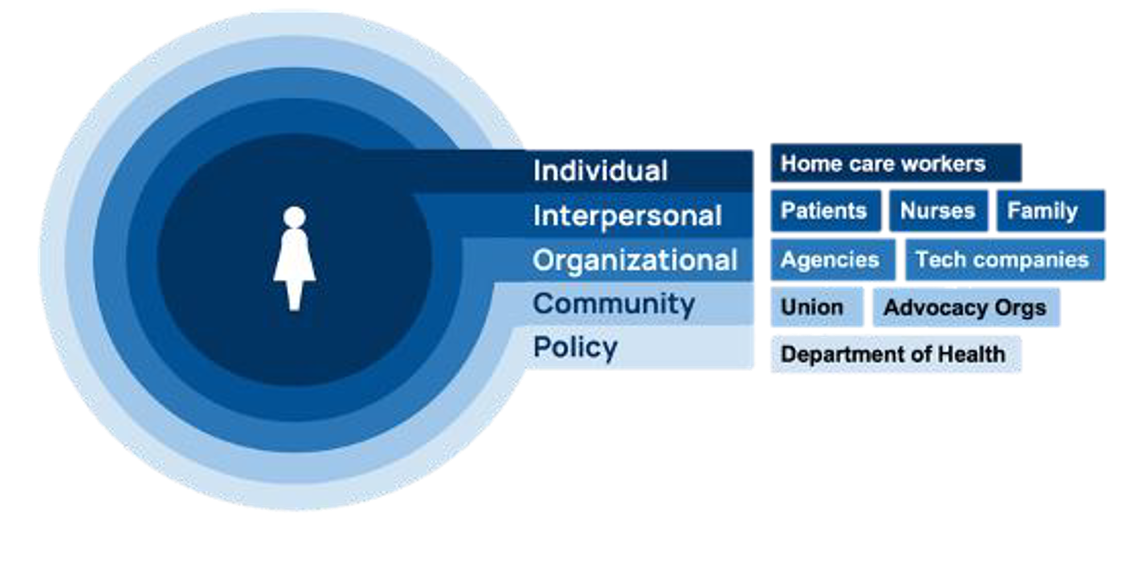
Conceptual Model based on the Social Ecological Model by Dr. Sterling (source)
To better understand the implications of current and near-future AI deployments in home care work, we conducted a qualitative study to understand how AI affects various stakeholders. Guided by the Social Ecological Model, we investigated the implications of AI in home care for workers, agencies, and advocacy groups.
Qualitative Method
We conducted 1-1 semi-structured interviews. We based discussions around 2 vignettes that outlined different uses of AI in the home care context to help place participant perspectives. We then synthesized qualitative findings from 22 interviews via structural coding of high-level topics, inductive generation of sub-codes within categories, and finally affinity mapping to get key themes.
Qualitative Data
We chose to use a qualitative method because we aimed to explore the perspectives, experiences and concerns stakeholders have regarding the deployment of AI in home care settings. A 1-1 interview approach enabled us to capture nuanced insights and understand the contexts, goals, concerns, and constraints each stakeholder had.
Recruitment
To recruit participants, we collaborated with a large healthcare union in the U.S., using purposive sampling for our particular target stakeholder groups. As these groups of participants were hard to reach, we also used snowball sampling -- asking participants to refer others.
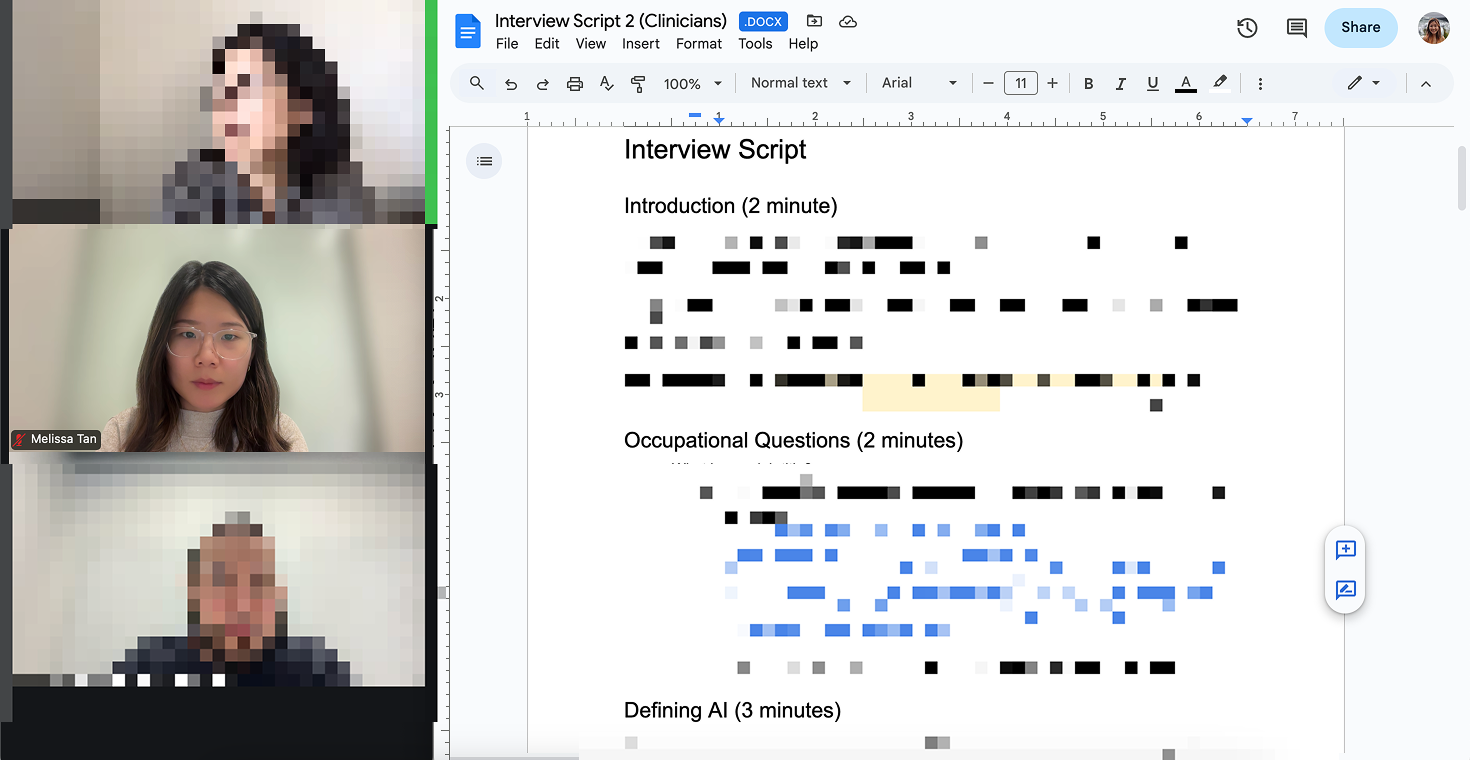
Clinician Stakeholder Interview
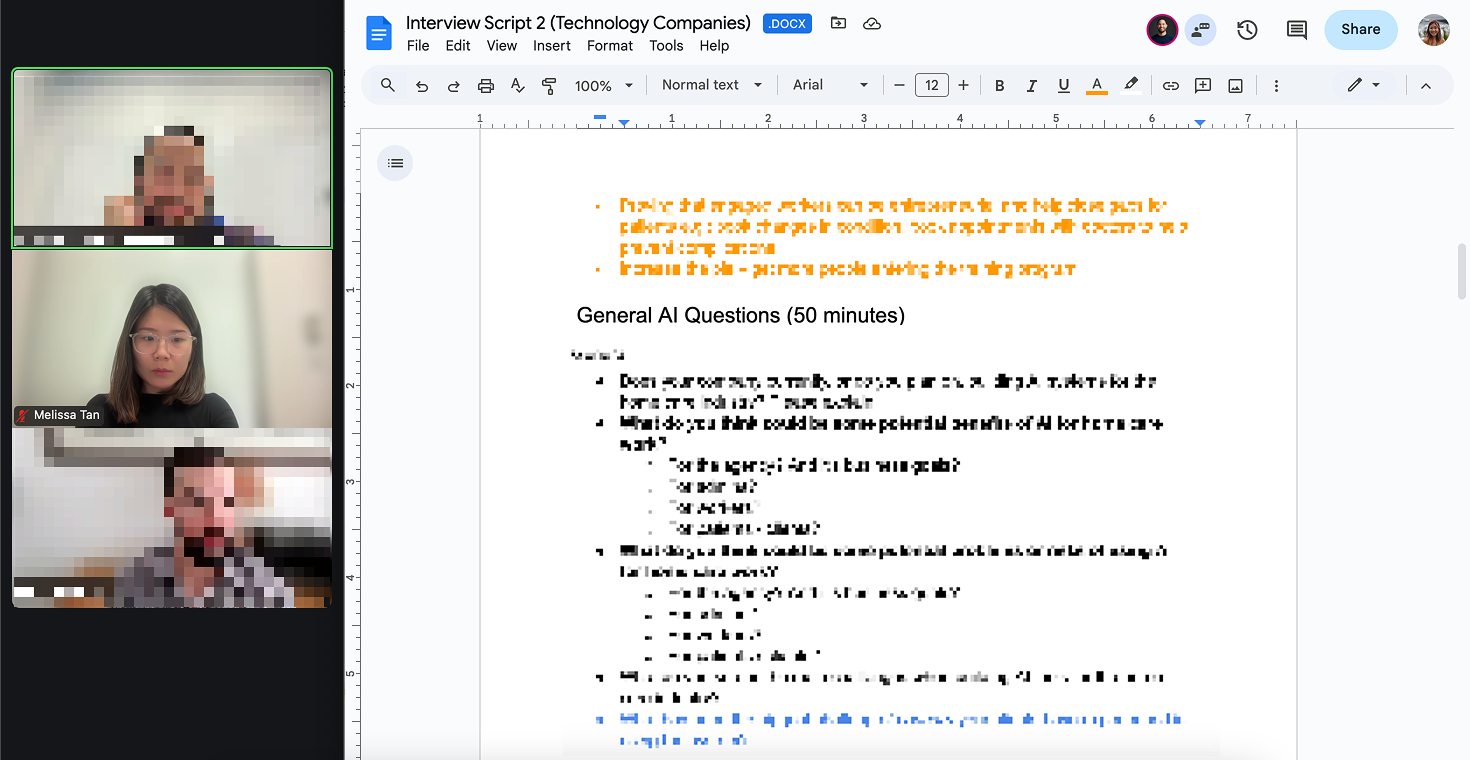
Tech Company Stakeholder Interview
Deductive and Inductive Coding Tree
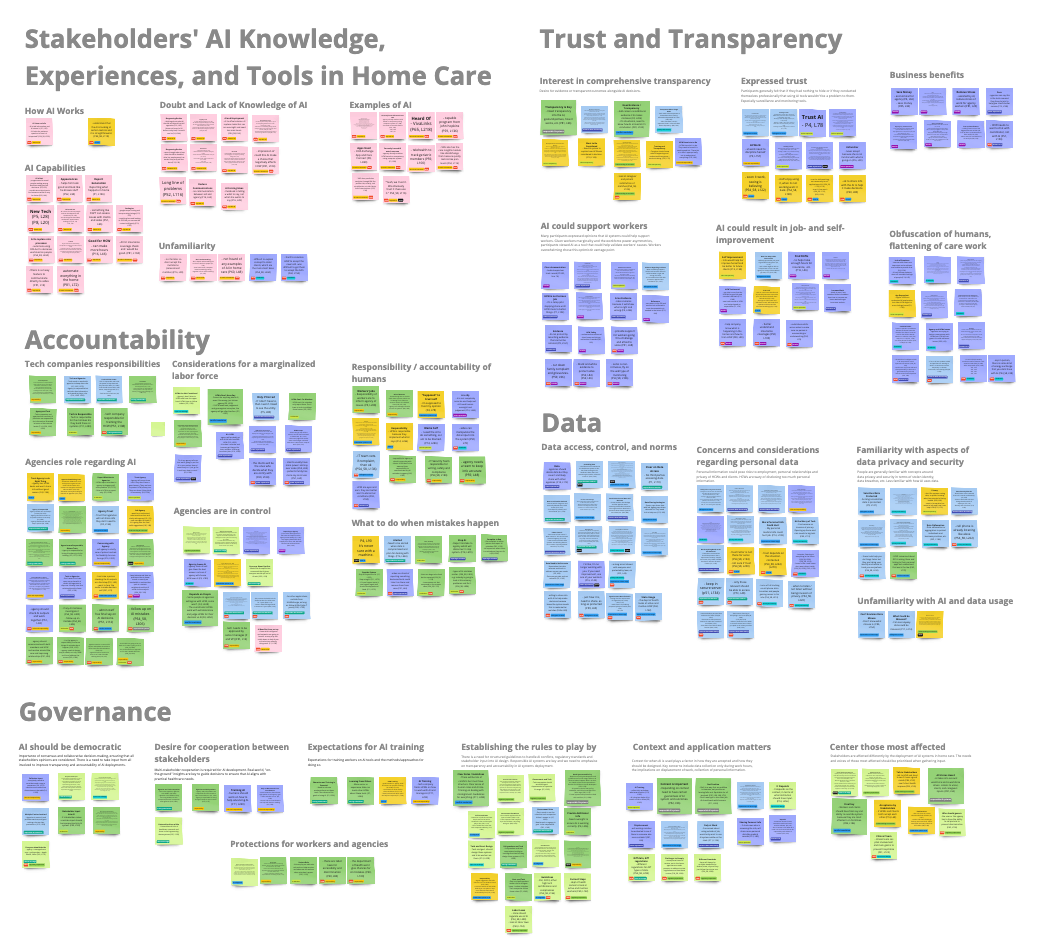
Affinity Mapped Themes
Findings
We found that HCWs generally lacked an understand of AI, and how it was already being used in their work. Although these tools may improve efficiency in home care, participants were concerned about the labor involved in keeping data accurate and updated. All stakeholder groups wanted more inclusive governance that engaged HCWs, agencies and advocates.
Discussion
Key Discussion Points
We first acknowledge the power imbalances and reinforcing systemic bias in home care. Workers risk being held responsible for AI mistakes, which may further isolate HCWs and burden them with unrecognized digital labor.
We also identified large variances in stakeholders' understanding of AI. There is a need to advocate for stakeholder-first literacy and education to help them understand and navigate the role of AI in home care. Efforts should prioritize enabling HCWs to recognize, contest, or contribute to decisions made by AI systems.
Next Steps
We are currently gathering perspectives from more stakeholder groups, namely technology companies and clinicians. We aim to compare our findings and publish our work in a peer-reviewed medical journal later this year.
Wearable Technology
Second author for accepted paper at CSCW 2025 (ACM SIGCHI conference on Computer-Supported Cooperative Work & Social Computing).
CSCW is the premier venue for research in the design and use of technologies to support collaborative work and life activities.
CSCW is the premier venue for research in the design and use of technologies to support collaborative work and life activities.
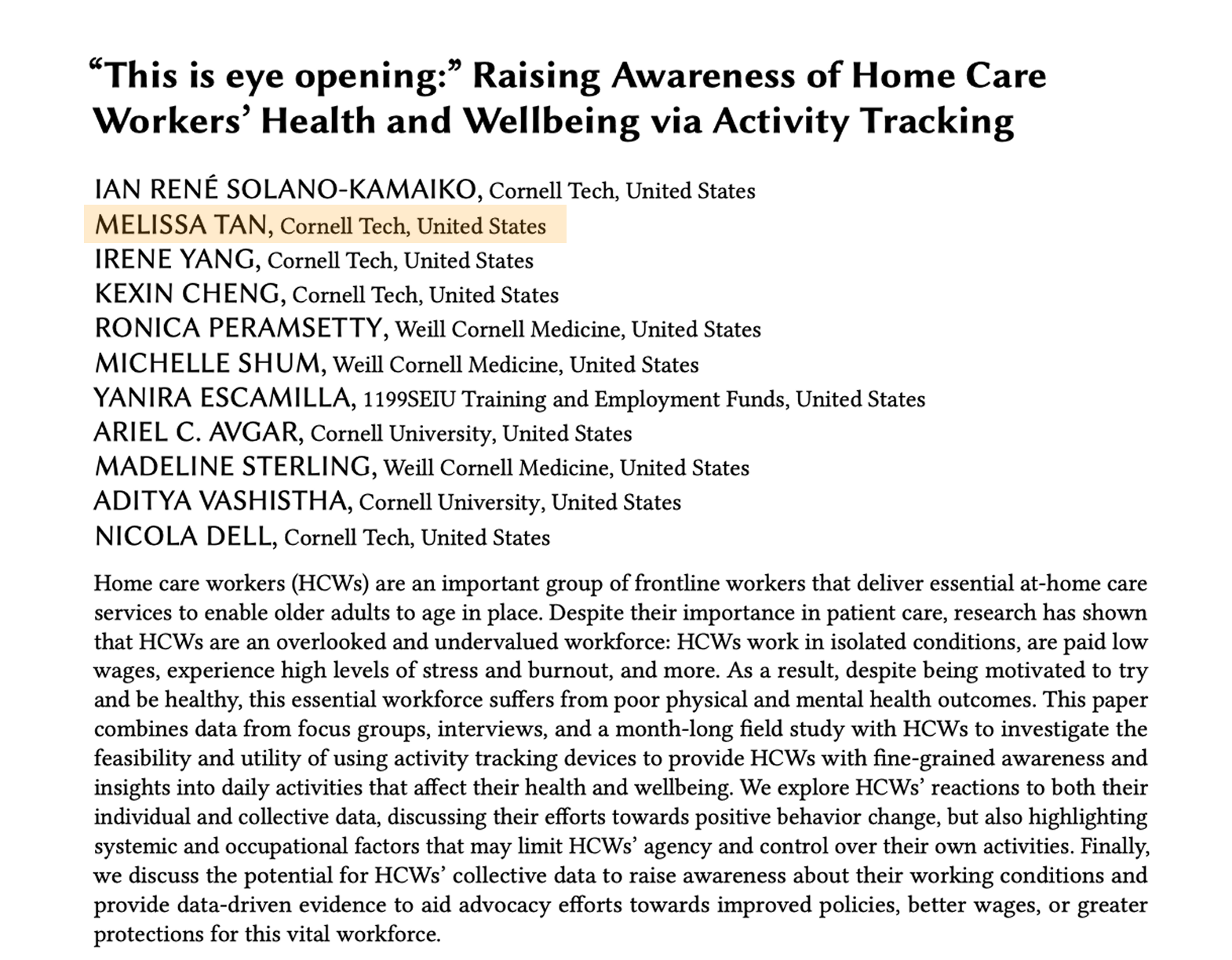
Field Study on Wearable Technologies for Home Care Workers (read here)
Summary
Expand for a pictorial overview of the Wearable Tech study.
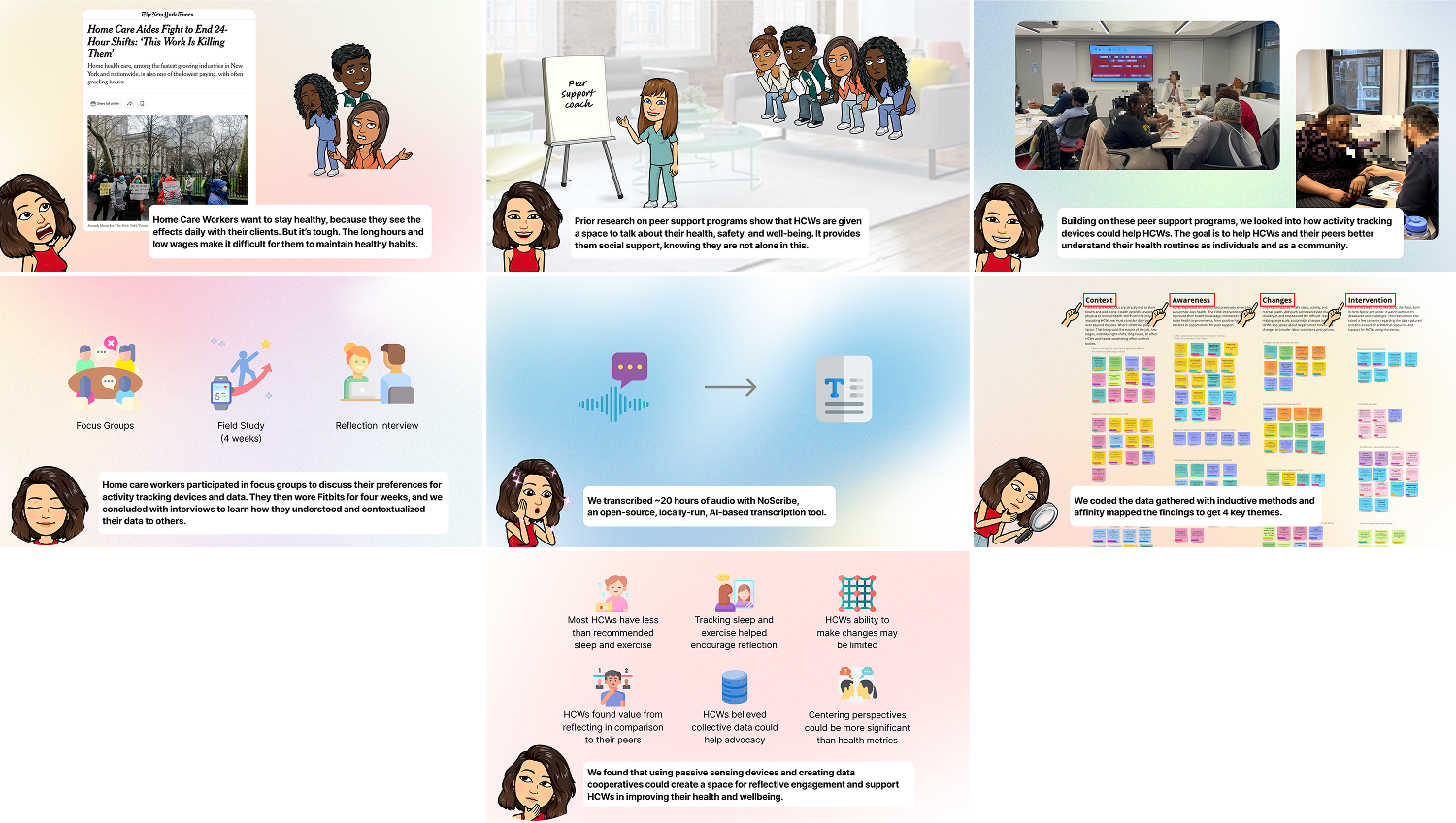
Story behind the wearables work.
Problem
Despite the challenges HCW face at work, research suggests they are motivated to be healthy, driven by their desire to care for others and their work with sick patients.
Research Questions:
- Do HCWs think tracking their health and wellbeing would be useful?
- Would HCWs’ be willing to track and share their data with others?
- What kinds of tracking devices might be appropriate for home care contexts?
We investigated the feasibility and utility of using activity tracking devices to provide HCWs with fine-grained awareness and insight into their daily activities that affect their health and wellbeing.
Visualization of HCWs Realizations on Their Work-Life Balance.
Mixed Method
We conducted mixed method research including in-person focus groups, interviews (remote and in-person), and a four-week field study with 17 participants, focusing on participants' sleep and activity metrics.
We chose to use a mixed method approach to contextualize the quantitative data within HCWs lived experiences and situate our findings within the complex work environments of HCWs.
We chose to use a mixed method approach to contextualize the quantitative data within HCWs lived experiences and situate our findings within the complex work environments of HCWs.
Quantitative Data
Quantitative data enabled us to obtain objective data on HCWs' sleep and activity to measure patterns and compare them against health standards.
Qualitative Data
Qualitative data enabled us to understand HCWs' perceptions of wearable technologies and how acceptable different forms of wearable technologies are. It also helped us uncover social, emotional and structural factors that affected HCWs' ability to make changes based on the data they see from their own personal tracking devices.
Study Process
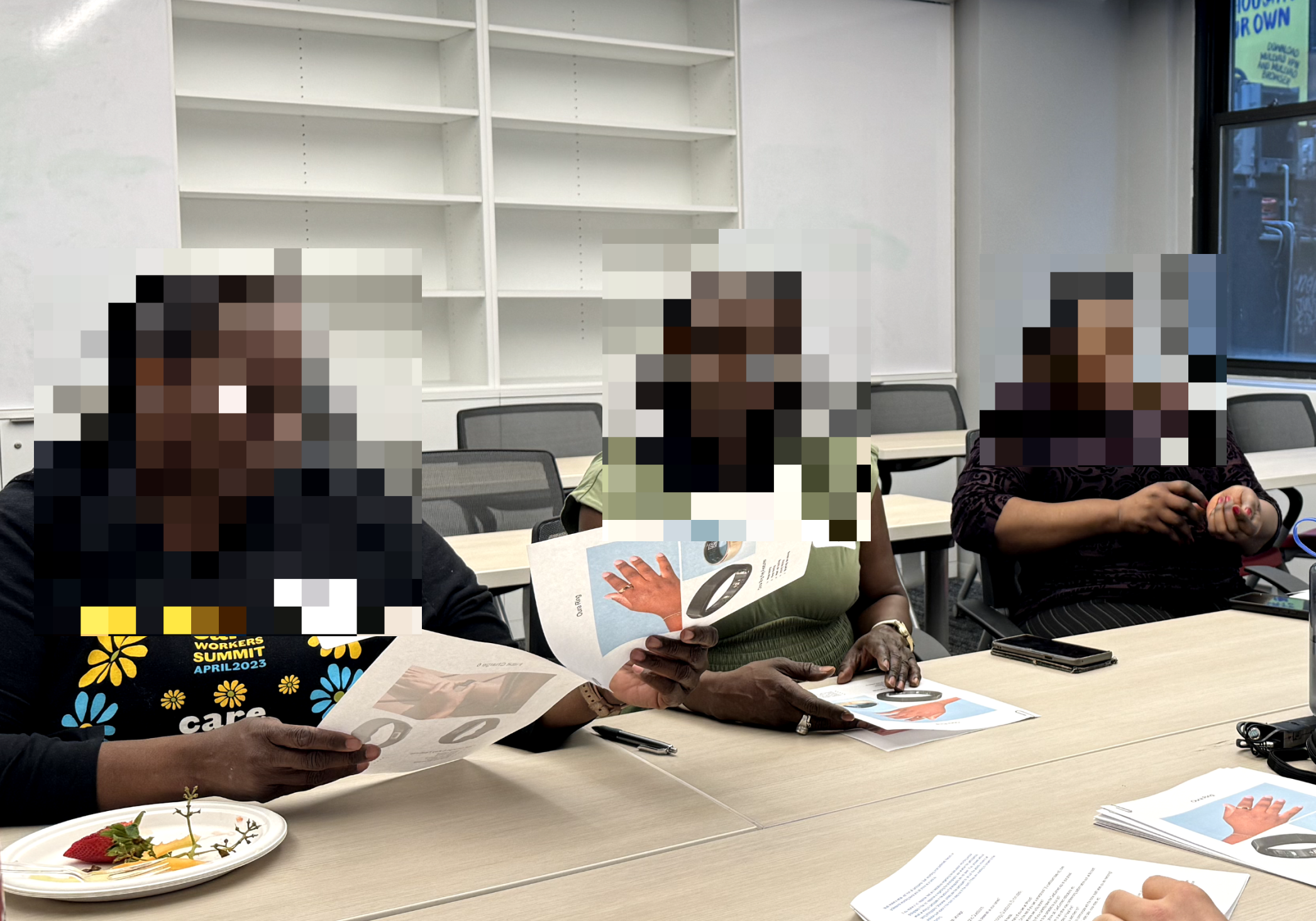
Focus Group

Participant Trying the Watch-based Wearable Device
To facilitate concrete conversations about participants' real data in relation to a group of peers, we collected each participants' data and developed personalized insights and visualizations. These visualizations featured minimal, pared-down designs that incorporated NIH recommendations and comparisons with peer data so participants could easily understand where they stood.
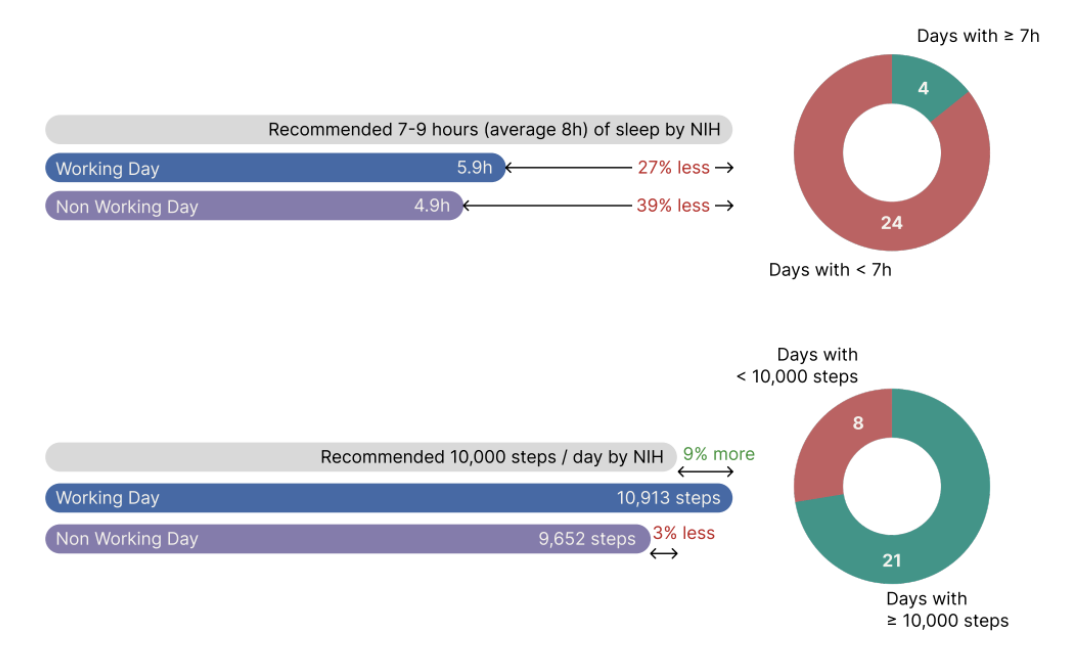
P12 Participant Data vs NIH Recommendations
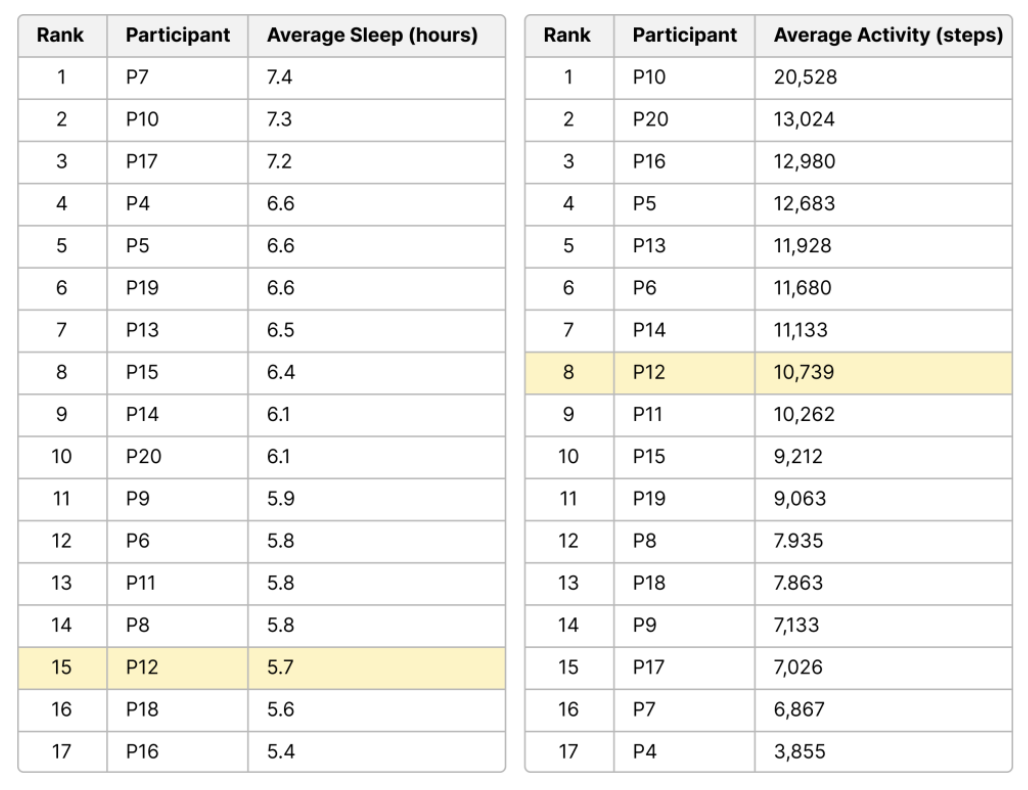
P12 Participant Data vs Peer Data
Findings
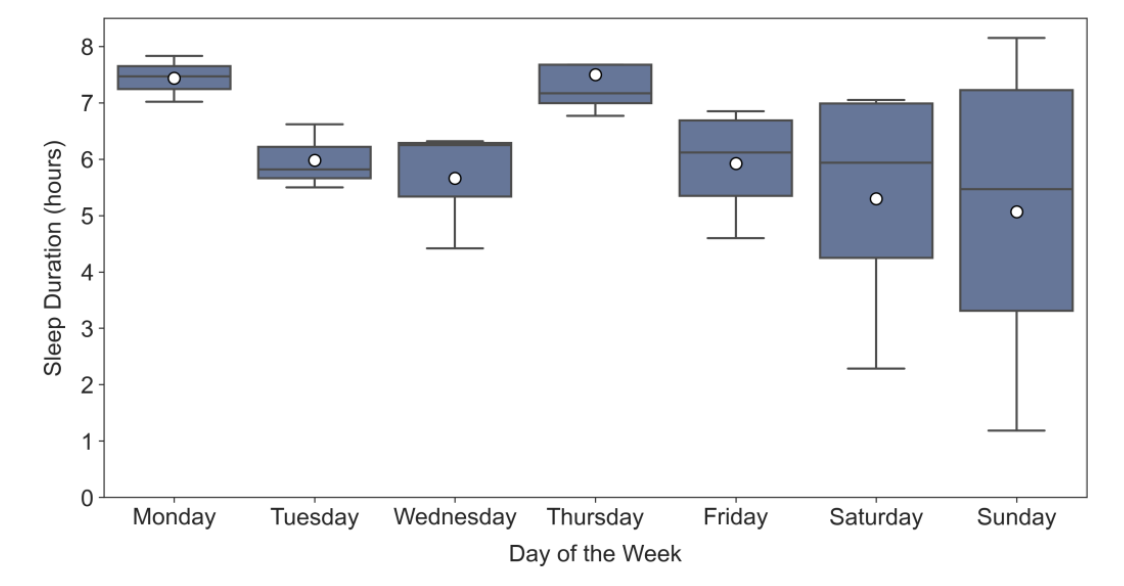
P20’s sleep duration across the four-week trial. Fridays, Saturdays, and Sundays correspond to the days that P20 worked overnight. The larger plot variance on these days indicates that P20 sleep was more variable.
We found that HCWs were surprised by their personal data. They often feel that they needed to choose between sleep, work, and exercise. Despite their desire to change, structural barriers like overnight shifts and erratic work schedules made it difficult.
Referencing collective data helped them contextualize their experiences while fostering togetherness, solidarity, and even friendly competition. Employing personal tracking interventions could offer support in positive health-related changes in HCWs, improving their wellbeing and by extension, the quality of care they provide to their pateints.
Referencing collective data helped them contextualize their experiences while fostering togetherness, solidarity, and even friendly competition. Employing personal tracking interventions could offer support in positive health-related changes in HCWs, improving their wellbeing and by extension, the quality of care they provide to their pateints.
Discussion
Key Discussion Points
Approaches that center HCWs' perspectives and experiences rather than simply showing health metrics may work better at depicting the lived realities of HCWs. For example, high step counts for HCWs often indicate labor intensity, instead of wellness.
Future work could explore data cooperatives to enhance HCW advocacy by highlighting the toll HCWs endure. Futhermore, data sharing programs amongst peers support programs could strengthen HCWs networks in the otherwise isolated workforce.
Next Steps
Working closely with Weill Cornell Medicine, we hope to submit to a peer reviewed medical journal in mid-April 2025.